“How do you know?” is the third question of the big three facing food processors as they conform to the requirements of the Food Safety Modernization Act (FSMA). Under FSMA, a food processor needs to know “What is your process?” as well as “Why is it your process?” With answers to these two questions, a food processor can turn their attention to the third question: “How do you know you did your process?” That is the focus of this article. Having answers to these three questions is not new for people interested in food safety. These questions are fundamental to food safety, even if they have not always been asked in this way. However, the standards for knowing are becoming ever more stringent.
For the most part, the food industry has responded to the challenges of knowing what has been done by adopting new technology as it has become cost effective or necessary to meet the expectations of the marketplace. Recognizing that one or both of these conditions has been met can greatly affect the future prospects of a company. Unfortunately, it is not always easy to recognize these situations when they arise. These changes have been both revolutionary and evolutionary. In many cases, the response has been to adopt greater automation and online instrumentation. The advent of inexpensive digital controls to replace complicated mechanical systems has caused many changes. As with most changes, there is peril in just relying on automated systems. The adage “trust but verify” is good advice. In this article, we are going to examine several somewhat generalized examples in which process controls that were once good enough have been replaced by new approaches.
In these examples, we will see that the process control parameters are not changing, but our ability to monitor and control them is changing. In some cases, there can be changes in the fundamental understanding of the process that create new opportunities for control. In all cases, if a primary process parameter is not controlled, a process will inherently be out of control and may yield undesirable results. Some control parameters are inherent to equipment design, but some forms of control are not apparent. It is often these parameters where control has evolved and less desirable control approaches are rejected.
A Mature Example for Perspective
Thermal processing is a good first illustration with a long history filled with examples. Thermal processing experienced its initial growing pains more than 2 centuries ago with the efforts of Nicolas Appert (1749–1841). Without primary records, I will postulate that Appert’s control para-meters included the maintenance of a boiling cooking kettle for temperature, cook time, bottle size and product. From these early beginnings, thermal processing has evolved from cooking in bottles and cans to a host of different cooking and packaging technologies. It is still evolving under pressure to deliver fresher, less-cooked products, pressure to fit into alternative packaging and all of the other economic pressures that a food processor faces to remain competitive. Nevertheless, the fundamental control parameters remain these same. The science associated with thermal processing has evolved and provided more sophisticated labels for these parameters as we leverage past knowledge to new situations. Process time replaces cook time because we want to properly account for heating and cooling time. We might have a holding tube instead of a package. We might have a hot-fill-and-hold process. Instead of bottle size, we might consider critical dimension to include spacing between heat exchanger plates or a variety of packaging types. With this list, we can turn our attention to some examples of the evolution of control.
Appert’s boiling kettle for temperature control has been replaced over time by thermometers, certified thermometers, thermistors and thermocouples. All of these are increasingly sophisticated tools for measuring temperature. Processors have gone from systems monitored intermittently by an operator to various flavors of automated logging systems, including chart recorders and computers. The shift to these new technologies has not been instantaneous. The new technologies were validated as being better or more robust. To ensure faster corrective actions, automated systems have been programmed to respond to small deviations to keep the process under control and divert underprocessed product back for more processing. Nevertheless, we can still find a contemporary use of Appert’s temperature control approach when jams, jellies and other fruit products are bottled using one of those once-ubiquitous blue porcelain canners on the stove. We can see that the situation can dictate the appropriate way to measure temperature for thermal processing. It is likely that the home canner does not even keep a paper record of the cook time.
Similarly, Appert’s cook time has been replaced, in some cases, by residence time in hold tubes at specific flow rates or by speed in continuous retorts of fixed lengths. Undoubtedly, some operations still do batches where time is monitored directly, but they certainly are not being timed by the bells of the church tower. Given a desire to minimize process time, it has become increasingly important to measure process times with precision. The ability to measure a short time is greatly impacted by the uncertainty of the timing device. Precision becomes a hugely important factor with ultrahigh-temperature processes where process times can be very short.
Clearly, the marketplace would accept Appert’s products only as a novelty at this point. The marketplace demands more sophisticated control of thermal processes. Additionally, there are regulations for low-acid foods that force particular types of control on processors. With the long history and evolution of thermal processing in foods, it is easy to see its adaptation to new technology. The standard for knowing the time and temperature of a process has evolved. Additionally, the standards for documentation have evolved so that if something goes awry, there are records to see whether the process failed or there was a failure to process properly.
Technology Has Changed How We Know
Today, we are experiencing the “Internet of things”—the embedding of networking technology into household appliances and consumer goods. Computers and digital control are pervasive. Increasingly, sensors are used for real-time analyses. Sensors provide high sensitivity, reproducibility, selectivity and mobility. They have a low cost of ownership and gradual replacement and/or parallel use when compared with complex and cumbersome analytical laboratory instruments. The reduced need for human involvement is also desirable, primarily to reduce error and time. Whether used “in-line” or as a “stand-alone,” the sensors can be integrated in conjunction with Wi-Fi technologies and used for real-time transmission of contamination alarms and/or test results to remote servers.
Sensors come in many types. There are many electrodes and probes. These devices respond to many different stimuli and are appropriate for diverse processes. The following list illustrates the diversity of these devices: thermocouples, smoke detectors, electronic noses, in-line indices of refraction, conductivity electrodes, mass flow meters, pressure transducers, etc. If there is money to be made, someone will figure out how to measure a particular stimulus or a surrogate. However, it is important to ensure that these alternative approaches will actually measure the desired stimuli in the actual working environment.
The challenge of the working environment can be illustrated with the use of an oxidation-reduction potential electrode to monitor chlorine. In a simple water system, this works reasonably well, but it rapidly breaks down when other materials are added to the water and the pH changes. If a sensor is not validated in the working environment, you cannot know if your process is under control.
The laboratory has also changed. There has been a proliferation of rapid methods based on immunochemistry and biotechnology such as PCR. Instruments have become more automated and powerful. If you want to measure it, you can. Modern instrumentation is connected to computers. We are beginning to see devices connected to smartphones. Recently, a near-infrared (NIR) spectrophotometer for consumer use entered the market. This NIR instrument was suggested as a tool for determining the ripeness of fruits and other good and bad characteristics of products. The impacts of all these changes can be seen in almost any modern process.
For example, the relatively recent adoption of automated color sorting marks an evolutionary step in process control. It was not that long ago that small products such as raisins, nuts or berries were sorted to a limited degree by individuals visually inspecting the product as it passed in front of them on a conveyor. Processors struggled with balancing the cost of inspectors and the value of more uniform products. Efforts were made to ensure that incoming lots were as consistent as possible. Today, there are online color sorting systems that use puffs of air to reject particular product pieces that fall outside the desired tolerances. It is amazing to watch these systems operate at speed and see the effectiveness of the sorting. The marketplace has come to accept this improvement in consistency. The standard for color consistency has evolved.
We can also see the evolution of process control in the systems for batching and controlling liquid blending for juices and other beverages. There are computer-controlled batching systems with mass flow meters. There are sensors to confirm that mixtures have the right density. There are automated systems for monitoring incoming water. These systems inherently document what was done, verifying that the process was followed. Paper records are still being used, but digital documentation is becoming the standard. These changes remove human intervention from repetitive tasks and reduce error, increasing confidence regarding knowing what was done.
Compounding of Errors
We must face a variety of errors in our attempts to know that we did our process. Three types of measurement error are especially troubling in that they can make out-of-spec product appear to be in spec. The first of these is observational error. Any time a measurement is made, an observation is obtained. This observation will deviate to some extent from the true value. The second type of error is calibration error. No measurement is more accurate than the reference. In fact, all measurements ultimately relate to some reference. And finally, there is the loss in accuracy over time, or drift.
Another family of error is the process target. There will be a range for the control parameters that yield the desired process if only because the process metrics have the three types of measurement error we just described. These ranges generally become the process specification, and anything outside of these ranges is out of spec.
The real trouble comes when Murphy intervenes and both types of error work against you. This is unlucky, as both types of error should be random. However, randomness necessarily includes all possible combinations. Under these conditions, if the errors are large enough, consumers may be at risk, depending on the hazards the process is meant to control.
A Developing Example
The science of food processing is not uniformly developed across all sectors of the food industry. We can and do leverage experience from one sector to another, but without a fundamental understanding, the tools we have been discussing only generate data and do not ensure process control. This is the situation in the value-added produce sector, particularly leafy green processing. Most leafy green washing processes have empirical data showing the product is largely safe and wholesome, but they are not really ready to address the three questions of FSMA. The base of fundamental research is increasing, but this is taking time to evolve and is not structured to work as a cohesive whole. The processes used by the various players are different. Multiple sanitizers, including chlorine, chlorine dioxide and peroxyacetic acid, are used in these processes. It is instructive to look at the gaps in our knowledge to see how efforts to know that the process was achieved are evolving.
The first step to having validated processes is understanding what must be achieved. In thermal processing, this was a simple question. We wanted a process sufficient to kill the more-than-expected population of organisms of interest. Leafy green processing does not have a kill step that can be expected to provide a 4- or 5-log reduction in Salmonella or pathogenic Escherichia coli, which would obviate the need for any other microbial control metric. Therefore, we need alternative metrics for establishing process validity. Three probable candidates include control of cross-contamination, lethality or log reduction and some measure of chemical safety. At this point, we are seriously hindered because we do not have a standard for any of these metrics. Many researchers have generated data, but there is no standard method for measuring cross-contamination. We do not have a standard method for measuring lethality on a leafy green that allows comparison among different processes and experiments.
Nevertheless, with these developing metrics in mind, we can turn our attention to the process parameters. Here again, the knowledge is limiting. For this article, we will focus on chlorine as the water sanitizer. Therefore, we can focus on chlorine concentration and pH as the primary control parameters. Other parameters have been suggested but will be ignored for this article, given their secondary importance.
Knowing the parameters to control is a good start, but one must also know to what level they need to be controlled. At present, there is still much disagreement about what level of chlorine is needed and what pH is necessary to provide an effective process. This is further complicated by the lack of agreement over how these simple parameters are measured and controlled, which brings us back to the focus of this article.
Addressing pH as the easier parameter first, pH probes are everyone’s choice. There was a time when pH test strips might have been considered viable options, but they are manual tools and generally lack the precision of a pH probe. These probes must be calibrated and readings must be collected. Most pH probes come with calibration procedures. Each processor needs to consider how often this must be done to ensure that readings are accurate. Nominally, calibration must be done often enough that there are no significant errors in the reading. A significant error is one that would allow out-of-spec product to be produced.
It may be tempting to use manual pH readings. This allows for cleaning of the probe and provides a check that the probe is performing normally. Such manual readings are inherently less frequent than an in-line probe that might be sampling every second and providing an average over a short interval. The problem with manual readings is that if an out-of-spec reading is generated, all of the product since the last reading within the tolerance would not have been produced under a valid process. Herein lies the rationale for continuous monitoring: It is possible to have a digital record documenting process performance.
Turning our attention to the monitoring of chlorine, there are several approaches. Operations using test strips tend to always have 10 ppm chlorine, even when they do not. There are a number of procedures based on the N,N-diethyl-1,4-phenylenediamine sulfate dye. These procedures can work well in clean water but are somewhat problematic in wash water with high organic loads. These tests are generally manual but can be automated. The manual nature of these tests presents the same problem as the manual pH method: There is a large amount of product produced that is out of compliance with the desired process if a reading is out of range. Both of these methods include both hypochlorite and hypochlorous acid in the free chlorine when only hypochlorous acid is the active form, giving an overstatement of the sanitizer present. Recently, probes that measure hypochlorous acid have entered the marketplace. These are coulometric and therefore subject to variability with flow. They consume chlorine as part of the measurement process. Additionally, they are affected by pH and must be calibrated at the working pH. Fortunately, these electrodes are not affected by organic load, making them very tolerant of the working environment.
Combining probes for both pH and hypochlorous acid, one can achieve statistical process control of both pH and chlorine, yielding a controlled process. If that controlled process achieves the desired metrics, the process can be validated and there is a good answer to the question “How do you know?”
Moral of the Story
As much as we might like to separate the three questions of FSMA, we are locked in a cycle where better understanding of one question leads to greater demands from the others. It is a cycle of continuous improvement, which is generally a good thing. In each cycle, we improve our answer to each question and ultimately provide better and safer products to our customers and consumers. That which is acceptable today may not be acceptable tomorrow. To stay in business, we need to be mindful of the opportunities to improve.
Eric Wilhelmsen, Ph.D., is an Institute of Food Technologists-certified food scientist, serving over 30 years in academic and industrial positions. He can be reached at the Alliance of Technical Professionals: eric.wilhelmsen@atpconsultants.com.
How Do You Know? The Evolution of Food Processing Technology
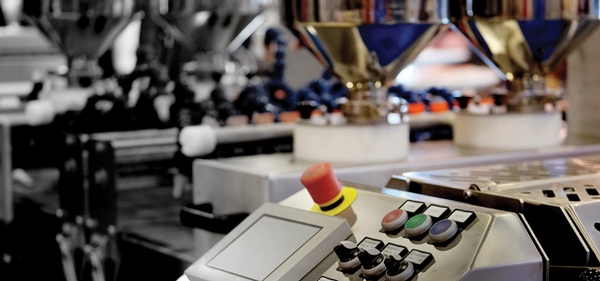